As part of supply chain management, it is important to conduct demand planning. Forecasting demands will help you prepare when those demands come in. You can avoid high operating costs and maximize profitability when you can balance inventory levels with demand.
However, traditional demand planning often fails to account for modern market dynamics, resulting in information gaps. Relying on such data will result in inaccurate forecasts. To avoid stockouts or overstocks, it is essential to have effective demand planning. This is where AI demand forecasting comes in handy.
Making complex real-time calculations is essential to maintaining optimal inventory levels and knowing what and when to order. For improved accuracy in forecasting, many businesses are now taking advantage of AI-powered tools. In this article, we’ll help you understand AI demand forecasting and how it can help with inventory planning to maintain optimal inventory levels regardless of market volatility.
What Is AI Demand Forecasting?
The integration of digital technology is becoming more common, along with the changes in inventory management. Demand-driven forecasting relies on order data to predict future inventory needs but falls short in an unpredictable market. Too many discrepancies from these occurrences can strain resources, and your business will sink quickly.
With AI-enabled demand forecasting, you can leverage AI to refine and optimize the process. It’s a holistic and integrated approach that focuses on past sales data along with offline and online market trends. With AI, data is not just collated; it is mined, identified, predicted, and revealed in a way that traditional models simply cannot do.
Benefits of AI Demand Forecasting
AI-driven inventory planning solutions provide several benefits. For one, you can avoid human and manual calculation errors. As a result, businesses can order the right amount of stock at the right time, regardless of seasonality, promotions, or supplier lead times.
A New Approach to Automation and Consolidation
As your data sources are integrated, AI can predict demand and automatically update purchasing recommendations accordingly. AI tools can help you predict future sales.
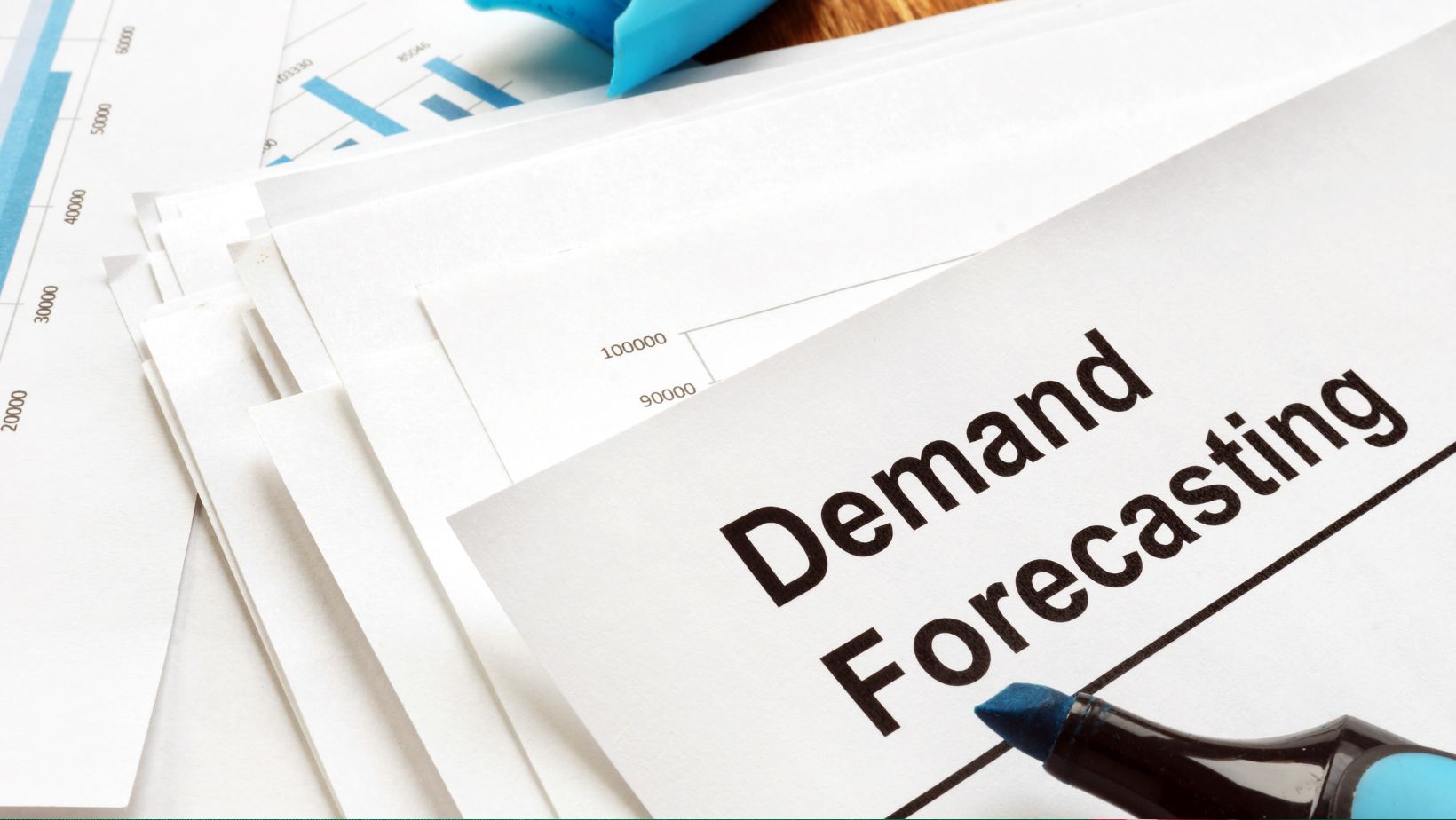
You can also design a restocking plan based on current stock levels and anticipated supply chain delays.
Managing the Unpredictable Nature of Suppliers
As supply chains become more complex and unpredictable, predicting and controlling their timing has become increasingly difficult. Previously reliable timeframes are now prone to frequent delays and deviations, which can easily disrupt inventory planning. AI tools can automatically apply updated lead time information to ensure ordering plans remain accurate and up-to-date.
Data Simulation for Historical Sales
You can use historical sales data to predict demand and put an effective ordering strategy in place. However, this is not possible for some products, such as new SKUs without sales history. A demand forecast model based on similar products can be simulated with advanced algorithms and artificial intelligence capabilities, automatically updated as new data is received.
Cutting Down on Overstocks and Stockouts
With AI tools, you can create custom forecasts by segmenting products, services, channels, and locations. Taking a variety of factors into account allows you to make specific ordering recommendations for every SKU in your catalog. This approach will help you keep your inventory at the right level and avoid unnecessary holding costs.
What Are the Disadvantages of AI Demand Forecasting?
Automated demand forecasting greatly benefits retail, e-commerce, and wholesale businesses; however, challenges exist. Below are some AI tools’ drawbacks:
- Dependability and quality of data. Data accuracy and relevance are essential for AI demand forecasting. Unreliable forecasts can be generated if the historical data used to train the AI model does not reflect current market conditions.
- Events and seasonal variations. Using pure AI models may result in inaccurate forecasts and inventory imbalances during significant market fluctuations, such as holiday seasons or unexpected events.
- Unexpected patterns. It may be difficult for AI models to recognize changes in consumer behavior that weren’t present in the training data. In the face of new market dynamics, this can lead to missed opportunities and incorrect forecasting.
- Maintenance is continuous. To remain relevant, AI models need regular updates and calibrations. Failure to update the AI models can degrade forecasting accuracy as market conditions change.
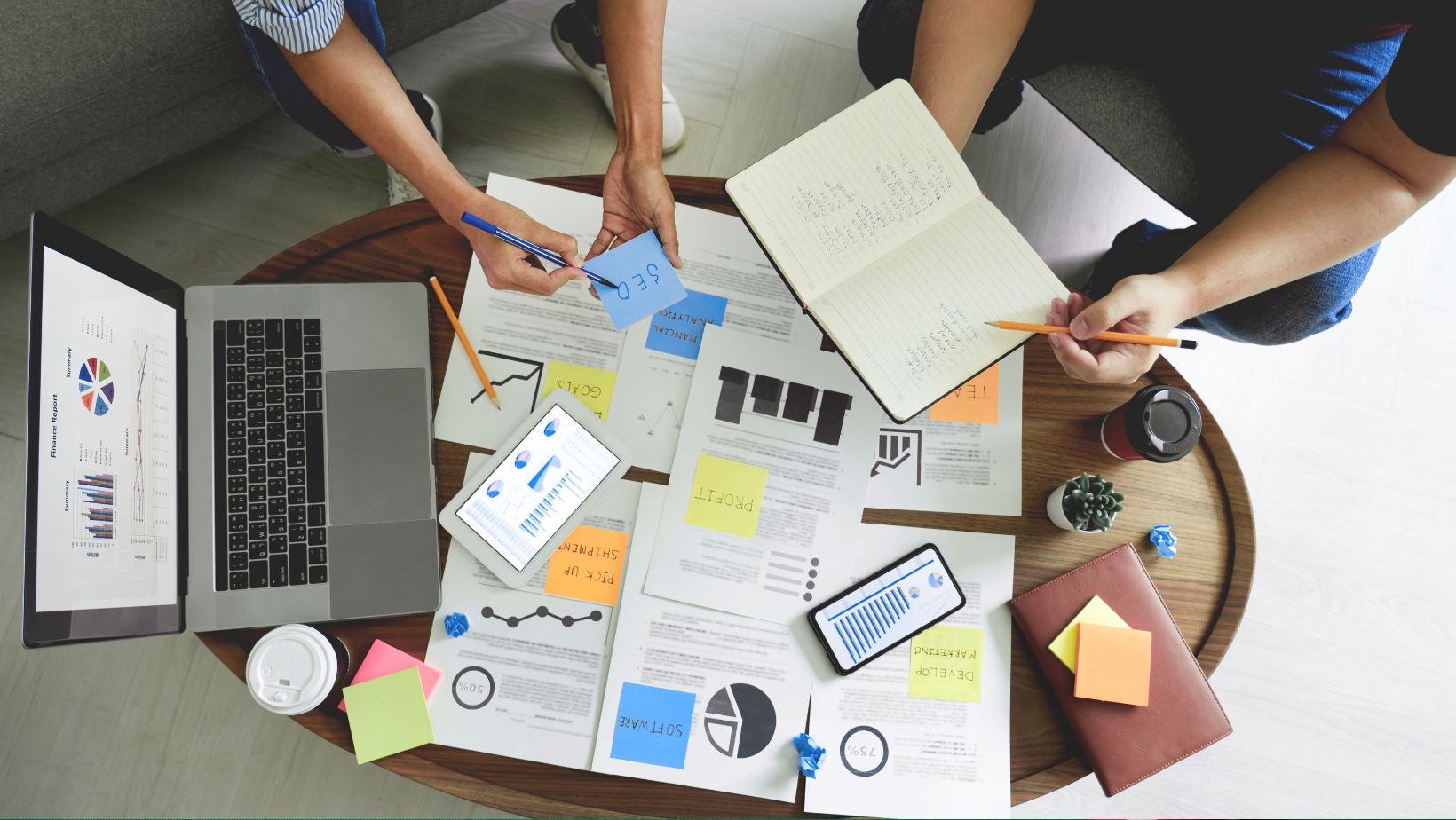
- The challenges of integration. Incorporating AI forecasting systems into legacy systems and processes can be complex and time-consuming. When data is not integrated properly, forecasting results can be inconsistent and contain discrepancies.
- Not enough customization options. Sometimes, AI forecasting solutions cannot be customized to fit specific business needs, resulting in generic forecasts that don’t match a business’s unique needs.
Empower Your Business With AI Demand Forecasting
In the modern business setting, learning how to leverage technology for success is crucial. With AI demand forecasting, you can eliminate guesswork and be more proactive in managing supplies and inventories. While AI forecasting is still in its early stages, it will undoubtedly be a powerful tool for businesses when it comes to reliable inventory planning.